|
Missing
Data
- Not all questions will be answered by all people, so missing
data is something which needs to be dealt with.
- There are various types of missing data:
- Questions which the respondent was not supposed to answer
(contingency questions)
- Not asked (interviews), missed, or unclear response
are another type of missing data.
- Questions which the respondent refused to answer (sometimes
hard to tell from missed in non-personally administered questionnaires.
- The "don't know" and "no opinion"
responses can be treated as missing data, but in some cases it
is better to leave them in the data as a separate category.
- You can give different codes to each type of missing data
if necessary, however each code should be clearly different than the
valid responses and valid codes for that question.
- Because it is easier to select one number for missing data
for all questions, the code(s) for missing data should be different
from a valid response for any question.
- Usually the number 0, 9, or 99 is used so that confusion
with valid data is avoided.
- Biases in the data can occur because of missing data. It
is important to check if a certain type of person did not answer a
particular question, which would skew the data. To check for biases,
you should cross-tabulate the people who did answer a question with
people who did not on other questions to see if patterns emerge.
- There are various ways to minimize the effect of missing
data from the sample:
- Delete all cases that have any missing data. This is
only useful if there are only a small number of cases which have
missing data, as deleting too many cases can lead to a greatly
reduced sample size.
- Delete the variable which is causing the non-response.
If there is one variable which has a lot of missing data, then
it can simply be discarded. This method only works if there is
one question which people refuse to answer and if that question
is not important to the study.
- Pairwise deletion uses a zero-order correlation matrix
to calculate the missing data in multivariate analysis. This method
uses similar cases to estimate the data. The problem with this
method is that it leads to a distortion of the data because if
a correlation already exists the estimation will be based on that
correlation, not the true values for a particular case.
- The mean approach simply takes the mean of the sample
and places it in for the missing data. A slightly more complex
method is to take certain background characteristics and calculate
the mean for that group. The groups must be selected on the basis
that they strongly correlate with the missing variable. The group
mean method gives more variability than the sample mean approach,
however it increases the correlation between the group characteristic
and the missing variable.
- Group -based random assignment can be used to maintain
variability. This technique takes the value of the previous case
(within the same group) for that variable and enters it for the
missing data. This eliminates the exaggeration of the relationship
between groups and variables and avoids loss of data all together.
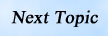 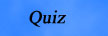
|