Samples
- Because you cannot survey the entire population you wish
to study, you must take a sample of that population.
- There are many ways that this sample can be done, however
the most important thing is that the sample is drawn representatively.
- Random or probability samples maximize the chances that
the sample will be representative.
- Random, or probability samples allow you to generalize your
data to the population which the sample was drawn from.
- Generalizing will be discussed in depth later.
- Although probability samples are not necessarily representative
of the population, by using random sample techniques you can perform
statistical analysis on the chances of the results are not representative.
- Probability sampling is based on the concept that everyone
in the population has an equal chance of being included in the sample.
- Sometimes researchers will oversample a sub-group
of the population to assure there will be enough respondents from
that group for statistical analysis.
- As long as the chances that a given respondent could
have been chosen for the sample are known, researchers can compensate
for unequal chances by weighting the statistical analysis before
generalizing to the population.
- The first step in finding a sample is to define the population
that you want to study.
- The population is important because this is who you can
generalize your findings to.
- You then must find a census, or sampling frame, which
is a list of the population.
- After you have a census, you must decide on the sample
size.
- Click
here for how large sample sizes should be. (See the
"samples" discussion on the Statistics page for a
discussion of factors that determine ideal sample size.)
- The final step in finding your sample is to decide on
a technique to randomly choose the sample.
Techniques:
- Simple random sampling (SRS) uses a random number list to
pick the sample.
- Each person in the population is given a number, and
those which correspond to the first X numbers (where X is your
sample size) in the random number list are chosen.
- For a sample random number list: click
here. (External link)
- The problem is that for many situations there is no
way to number (or even know) your entire population, so in many
cases this technique will not work.
- Systematic sampling uses a similar list of your population;
however you do not need a random number table. For systematic sampling,
you:
- Take the total number in your population.
- Decide on a sample size.
- Calculate the fraction of total population over sample
size.
- Pick a number between 1 and the previous fraction.
- Use that number as your first person in the sample.
- Use the sampling fraction to select every case, so if
your fraction was 3 then select every 3rd person.
- For a walk-through: click
here.
- Not only do you again encounter the problem of listing
your entire population, but here the repetition of selection can
introduce biases. An example would be if the list was composed
of alternating male and female names, an even sampling fraction
will produce only people of one sex.
- Stratified sampling uses groups of people to guarantee representitiveness
or make sure that there are at least a certain amount of a certain
group in your sample.
- This technique is useful when you wish to look at small
portions of a population which may be excluded in a simple random
sample.
- For example, if you wanted to compare Native Americans
to the Anglo population of Colorado, you would have to use stratified
sampling to have enough Native Americans for comparison.
- To make a stratified sample, you select the groups which
you want represented and take individual samples from those groups.
- This allows you to determine what percentage of each
group ends up in your overall sample.
- Cluster sampling uses multiple stages of samples to address
the problem of a dispersed population or a population which would
be impossible to list. Click
here for an example of cluster sampling.
- Clusters are temporarily treated as sampling units,
but contain the final sampling units within them.
- First, clusters are randomly sampled from the entire
population which you want to study.
- Depending on the stages in your method, you perform
another random sample of smaller clusters within the selected
larger clusters.
- Finally, the elements are randomly samples from within
the smaller clusters selected.
- This method vastly reduces costs associated with travel
for dispersed populations, but it also can introduce problems
with representitiveness.
- The problem with simple clustering as shown above is that
it does not work for clusters which are not the same size.
- This is because while every cluster has the same chance
of being selected, elements within large clusters have a greatly
reduced chance of being selected in the final sample.
- Using the probability proportionate to size (PPS) technique
corrects this error.
- PPS takes into account the differences in cluster size
and adjusts the chance that clusters can originally be picked.
- This is done by stacking the odds toward larger clusters.
- Random digit dialing is a technique used over the phone
to get a random sample.
- Because there are problems with using telephone books
(people not listed, you want to include the entire US population,
etc.) computers can randomly dial numbers in pre-selected area
codes and exchanges (which can be already randomly selected).
- The problem is that only residential numbers are good,
so it can be frustrating to sit there while the computer dials
disconnected and business numbers.
- Links to further reading
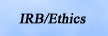 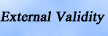
|